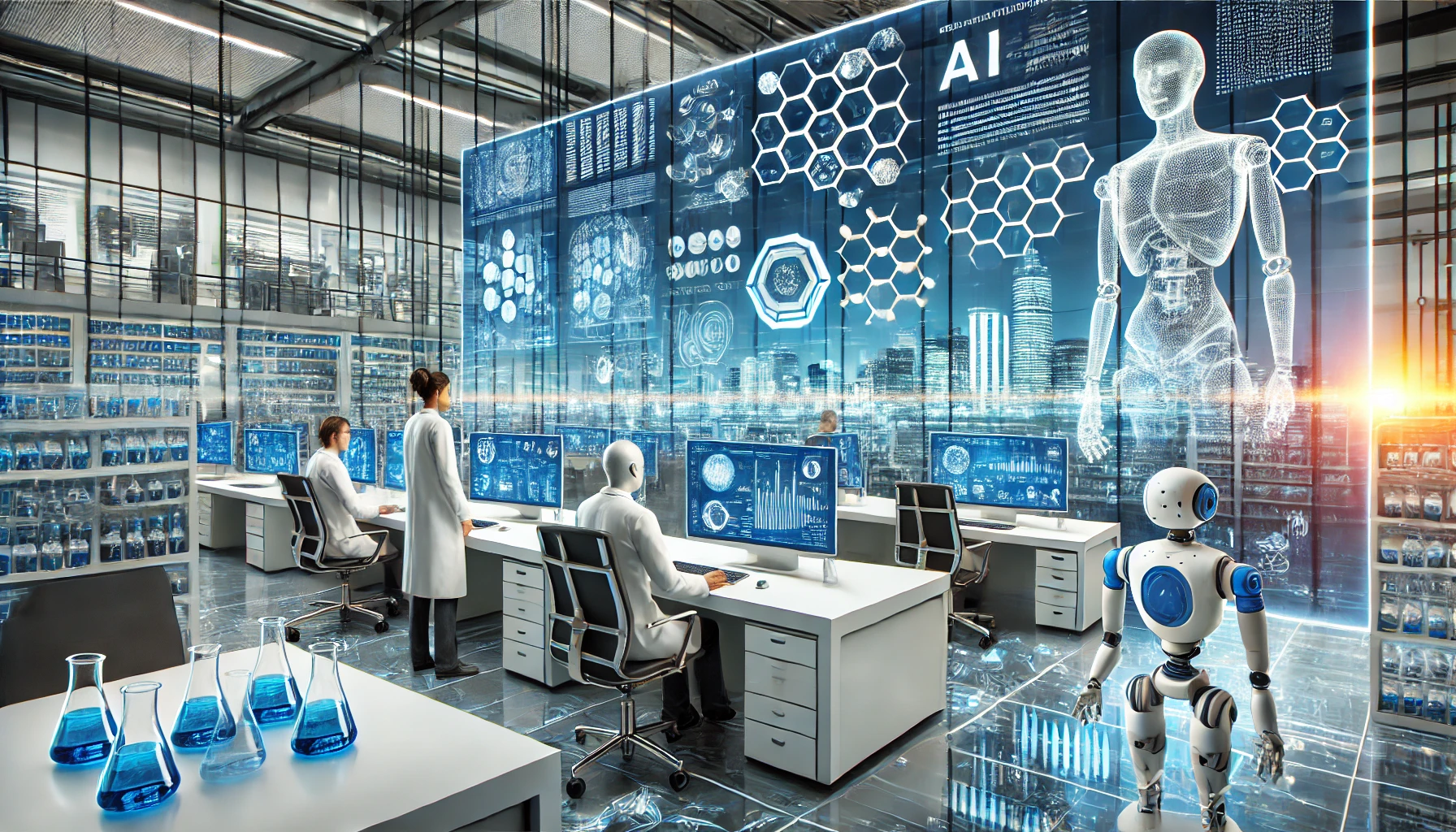
Drug discovery has long been a slow and expensive process. It takes, on average, 10 to 15 years and billions of dollars to develop a new medication from initial research to regulatory approval. The process involves multiple phases, beginning with identifying potential drug compounds, followed by extensive laboratory testing, preclinical studies in animals, and multi-phase clinical trials in human participants. Each step is crucial for ensuring safety and efficacy, but the sheer volume of data that must be analyzed—ranging from genomic information to clinical trial outcomes—creates significant bottlenecks. Many promising drug candidates fail during clinical trials, often due to unforeseen side effects or lack of effectiveness, further increasing costs and delays.
One of the biggest challenges in drug development is the complexity of diseases themselves. Many conditions, such as cancer, neurodegenerative disorders, and rare genetic diseases, have intricate biological mechanisms that are not fully understood. Traditional drug discovery relies on labor-intensive methods, where researchers manually test thousands of compounds in the hopes of finding one with therapeutic potential. This process is slow, expensive, and highly uncertain. Even after a drug candidate is identified, ensuring that it can be safely administered to humans and effectively target the disease remains a time-consuming endeavor.
However, artificial intelligence (AI) and big data are now transforming this landscape, making drug discovery faster, more efficient, and more accessible. AI can rapidly analyze vast datasets, including genomic sequences, molecular structures, electronic health records, and past clinical trial results, to identify patterns and predict which drug candidates are most likely to succeed. Machine learning models can simulate how different molecules will interact with human cells, drastically reducing the need for costly trial-and-error experimentation in labs. AI-powered drug discovery platforms can also repurpose existing drugs for new uses—an approach that proved valuable during the COVID-19 pandemic when researchers leveraged AI to identify potential antiviral treatments in record time.
Beyond identifying promising compounds, AI is also optimizing clinical trial recruitment, one of the biggest hurdles in drug development. Recruiting the right patients for a trial is often slow and inefficient, leading to delays and increased costs. AI-driven platforms, such as MyTrials.ai, are tackling this challenge by streamlining the matching process, ensuring that clinical trials enroll eligible patients more quickly. By making trial participation more efficient, AI is revolutionizing not just drug discovery but also how we bring life-saving treatments to patients who need them most.
The Power of AI in Drug Discovery
Traditionally, researchers relied on manual experimentation and trial-and-error approaches to identify promising drug candidates. This was an incredibly time-consuming task, requiring years of work to analyze chemical structures, understand disease mechanisms, and test drug interactions. Researchers would often screen thousands of chemical compounds in laboratory settings, conducting extensive biochemical assays to determine which molecules had therapeutic potential. Even when a promising candidate was identified, further refinement and testing were required to improve its safety, effectiveness, and bioavailability—how well the drug is absorbed and utilized by the body. This painstaking process meant that only a fraction of tested compounds made it past early research stages, with many failing due to unforeseen toxicity or poor performance in living organisms.
AI, however, is revolutionizing this process by processing millions of data points in mere minutes, identifying patterns and potential solutions far more quickly than humans ever could. Instead of manually sifting through massive datasets, machine learning models can rapidly analyze chemical structures, genetic markers, and biomedical literature to predict which compounds have the highest likelihood of success. These models use complex algorithms to recognize patterns in data that might indicate how a drug will interact with a disease target, significantly reducing the time needed for initial drug screening. Moreover, AI-powered virtual screening techniques enable researchers to simulate drug interactions computationally before physical testing even begins, further accelerating the discovery process.
One of the most groundbreaking examples of AI in drug discovery is DeepMind’s AlphaFold, which has revolutionized protein structure prediction—a crucial step in developing new treatments. Understanding how proteins fold and interact with other molecules is essential for designing drugs that can effectively target diseases. Previously, predicting protein structures was a slow and expensive process, often requiring years of experimental work using techniques like X-ray crystallography and cryo-electron microscopy. AlphaFold has changed this by using AI to accurately predict protein structures within hours, allowing researchers to map out disease-related proteins with unprecedented speed and precision.
Beyond protein structure prediction, AI-driven platforms are also enhancing drug repurposing—the process of identifying new uses for existing medications. By analyzing vast datasets from past clinical trials and real-world patient outcomes, AI can detect unexpected therapeutic effects of drugs that were originally developed for other conditions. This approach proved invaluable during the COVID-19 pandemic when researchers leveraged AI to quickly identify existing drugs, such as dexamethasone and remdesivir, that showed promise in treating severe cases. By repurposing known compounds, drug development timelines can be drastically shortened, reducing the need for early-stage research and safety testing.
AI is also transforming how researchers predict a drug’s safety and effectiveness before it reaches human trials. Traditional drug development often encounters roadblocks when a promising compound shows adverse side effects in later stages, leading to costly failures. AI-powered models can now simulate drug interactions in the human body, analyzing potential side effects and predicting how different patient populations will respond. By using real-world health data, such as electronic health records and genomic sequences, AI can help pharmaceutic
In short, AI is not only making drug discovery faster and more efficient but also reducing risk and improving the success rates of new treatments. With AI’s ability to analyze massive datasets, simulate drug interactions, and predict therapeutic outcomes, we are entering a new era of precision medicine—one where the right drugs can be designed, tested, and delivered faster than ever before.
Predictive Modeling in Clinical Research
Beyond drug discovery, AI is also reshaping clinical research through predictive modeling, a powerful technique that uses machine learning and big data to forecast outcomes in clinical trials. Clinical trials represent the most expensive and time-consuming phase of drug development, often taking several years and costing hundreds of millions of dollars. However, a significant portion of these costs stems from inefficiencies such as slow patient recruitment, poor trial design, and unforeseen adverse reactions. Many trials fail not because the drug itself is ineffective, but because of avoidable issues such as selecting the wrong patient population or encountering unexpected safety concerns too late in the process. AI-driven predictive modeling helps address these challenges by analyzing historical trial data, real-world patient information, and biomedical research to make smarter, data-driven decisions before a trial even begins.
One of the most impactful ways AI improves clinical research is by optimizing patient selection. Traditional clinical trials often rely on broad inclusion and exclusion criteria, leading to inefficient participant selection and high dropout rates. AI can change this by predicting which patients are most likely to respond positively to a new treatment based on their genetic markers, medical history, and lifestyle factors. For instance, an AI model analyzing genomic data might identify a subgroup of patients with a specific mutation who are more likely to benefit from a targeted cancer therapy, allowing researchers to tailor trials more effectively. This precision medicine approach not only increases the likelihood of trial success but also helps bring effective treatments to market faster by eliminating unnecessary participants who may not respond well to the drug.
Beyond patient selection, AI-powered predictive modeling is also revolutionizing trial safety and monitoring. One of the biggest risks in clinical trials is discovering serious side effects too late, leading to costly failures and even potential harm to participants. AI can proactively detect safety concerns by analyzing vast amounts of real-world health data, including electronic health records (EHRs), social media reports, wearable device data, and past trial results. For example, if a particular drug has shown subtle but concerning side effects in early-phase trials or in post-market surveillance, AI can flag these signals early, allowing researchers to refine the study design, adjust dosages, or even modify the drug formulation before progressing to later trial phases. Furthermore, wearable health technologies—such as smartwatches and fitness trackers—generate continuous patient data, providing AI with real-time insights into heart rate, blood pressure, and other key health indicators. This real-time patient monitoring enables earlier intervention and prevents severe adverse events from escalating.
Pharmaceutical companies and research institutions are rapidly adopting AI-driven insights to streamline trial designs, minimize costs, and improve overall success rates. AI allows for adaptive trial designs, meaning protocols can be adjusted dynamically based on real-time data rather than relying on rigid, pre-set guidelines. If early trial data suggests a particular dosage is more effective or that a certain patient subgroup is responding better, AI can help modify the trial in real time, reducing wasted resources and increasing efficiency. The result is not only faster drug development but also safer and more effective treatments for patients. By leveraging predictive modeling, the clinical research industry is shifting away from inefficient, one-size-fits-all methodologies toward personalized, data-driven trial strategies that prioritize both patient safety and scientific progress.
How MyTrials.ai Is Leveraging AI for Smarter Clinical Trial Matching
Despite these advances, one major challenge remains: finding the right patients for clinical trials. Patient recruitment is one of the biggest bottlenecks in clinical research, with up to 80% of trials experiencing delays due to enrollment issues. MyTrials.ai is tackling this problem head-on by using AI to match patients to clinical trials more efficiently.
By analyzing patient demographics, medical conditions, and clinical trial requirements, MyTrials.ai ensures that eligible participants find trials suited to their specific health needs. Unlike traditional recruitment methods that rely on manual screening and generic outreach, MyTrials.ai uses AI-driven algorithms to quickly filter through vast patient data and identify those who meet trial criteria. This not only speeds up the recruitment process but also improves trial diversity and accessibility.
Additionally, MyTrials.ai prioritizes patient-centricity, making the trial search and application process more transparent and user-friendly. Through intelligent automation, the platform reduces administrative burdens for both patients and researchers, allowing for faster trial enrollment and improved retention rates. As a result, clinical trials can progress more smoothly, bringing new treatments to market sooner.
A New Era in Drug Development
AI and big data are fundamentally reshaping the drug development landscape, turning what was once a slow, expensive, and uncertain process into a more efficient, data-driven endeavor. The integration of artificial intelligence into drug discovery and clinical research is not just about speeding up processes—it is also about making smarter decisions, reducing failures, and improving patient outcomes. With the ability to analyze vast datasets in ways that were previously impossible, AI is transforming how pharmaceutical companies, research institutions, and healthcare providers identify promising drug candidates, predict patient responses, and optimize clinical trial designs. This shift is dramatically reducing the costs, risks, and time associated with bringing new treatments to market.
By eliminating trial-and-error inefficiencies, AI-driven drug discovery platforms can rapidly screen billions of molecular structures, simulate their interactions with biological targets, and pinpoint the most promising candidates in a fraction of the time it would take using traditional methods. Machine learning models continuously improve as they ingest more data, leading to increasingly accurate predictions and better-informed decision-making in both preclinical and clinical research. Furthermore, AI-powered predictive modeling and real-world data analysis are enabling researchers to identify safety concerns earlier, personalize treatments, and design more adaptive clinical trials—reducing the likelihood of failures and ensuring that effective therapies reach patients sooner.
As AI-driven tools continue to evolve, the drug development process will become even more streamlined and precise. Future breakthroughs could include fully autonomous drug discovery pipelines, where AI not only identifies potential compounds but also designs and refines them, predicts their effects, and automates lab testing through robotics. In clinical research, AI could help create real-time, patient-specific treatment protocols, enabling an era of truly personalized medicine where therapies are tailored to an individual's unique genetic and biological makeup.
Companies like MyTrials.ai are at the forefront of this transformation, ensuring that life-saving treatments reach patients faster than ever before. By leveraging AI to match patients with clinical trials more efficiently, MyTrials.ai is helping to eliminate one of the biggest bottlenecks in clinical research—slow and inefficient patient recruitment. This not only accelerates trials but also improves access to experimental treatments for patients who need them most.
The impact of AI in medicine extends far beyond simply increasing speed; it is making healthcare smarter, more precise, and more accessible for all. As these technologies continue to advance, they hold the potential to revolutionize patient care, ensuring that groundbreaking treatments reach the right patients at the right time—reshaping the future of medicine as we know it.